NISER 081107 ID
Contents
NISER Wiki Resource Prototype - First template for a multi-disciplinary NISER activity
Summary
Visualization, understanding and interpreting real data may be challenging because of noise in the data, data complexity, multiple variables, hidden relations between variables and large variation. This NISER activity demonstrates how to use free Internet-based IT-tools and resources to solve problems that arise in the areas of biological, chemical, medical and social research.
Goals
This NISER activity has the following specific goals:
- to demonstrate the typical research investigation pipeline - from problem formulation, to data collection, visualization, analysis and interpretation;
- to illustrate the variety of portable freely available Internet-based Java tools, computational resources and learning materials for solving practical problems;
- to provide a hands-on example of interdisciplinary training, cross-over of research techniques, data, models and expertise to enhance contemporary science education;
- to promote interactions between different science education areas and stimulate the development of new and synergistic learning materials and course curricula across disciplines.
Motivation/Problem
Mercury contamination of edible freshwater fish poses a direct threat to human health. Largemouth bass is a fresh water fish that was studied in 53 different Florida lakes to examine the factors that influence the level of mercury contamination. Water samples were collected from the surface of the middle of each lake in August 1990 and then again in March 1991. The pH level, the amount of chlorophyll, calcium, and alkalinity were measured in each sample. Also, samples of fish were taken from each lake with sample sizes ranging from 4 to 44 fish. The age of each fish and mercury concentration in the muscle tissue was measured. Since fish absorb mercury over time, older fish will tend to have higher concentrations.
Data
Largemouth bass were studied in 53 different Florida lakes to examine the factors that influence the level of mercury contamination. Water samples were collected from the surface of the middle of each lake in August 1990 and then again in March 1991. The pH level, the amount of chlorophyll, calcium, and alkalinity were measured in each sample. The average of the August and March values were used in the analysis. Next, a sample of fish was taken from each lake with sample sizes ranging from 4 to 44 fish. The age of each fish and mercury concentration in the muscle tissue was measured.
Challenge
To make a fair comparison of the fish in different lakes using a regression estimate of the expected mercury concentration in a three year old fish as the standardized value for each lake. Determine the age of the individual fish in some lakes and correlate this with the average mercury concentration of the sampled fish.
Florida has set a standard of 1/2 part per million as the unsafe level of mercury concentration in edible foods. 45.3% of the lakes exceed this level. The smallest level of mercury concentration that the measuring instrument can detect is 40 parts per billion. Any level below that was set to 40 parts per billion. This, of course, "flatens out" the slope of the relationship at the low end as well as affecting the standardized values. These observations are usually on young fish.
Logarithmic transformations on some of the variables may provide insights into the relationships among the other variables in the study. For instance, alkalinity level may be associated with mercury concentration, and may help account for the higher levels of mercury.
Methods & Approaches
We now discuss the models & strategies for data understanding, inference and solving this problem and discuss checking/affirming underlying assumptions.
Physics
- What is an atom and what is the atomic structure of mercury?
Biology
- Why is mercury (and other heavy metals) accumulating in mussle tissue?
- What causes the toxicity of larger than normal amounts of mercury in the body?
- The Chlorophyll molecule.
- 3D structure of Chlorophyll using PDB/JMol viewer.
Chemistry
Engineering
Mathematics
- Fit models to some of the variables in this dataset.
Statistics
- Are there associations in these data between Alkalinity, pH, Calcium and Chlorophyll?
- What is the distribution of the average amount of mercury in the entire sample?
- Is there evidence of statistical differences between the two groups (according to the age_data variable) in either Alkalinity or pH?
Computational Resources
Internet-based SOCR Tools (including offline resources, e.g., tables).
Some exploratory data analyses (EDA plots)
- Scatter plot (Lakalinity vs. pH)
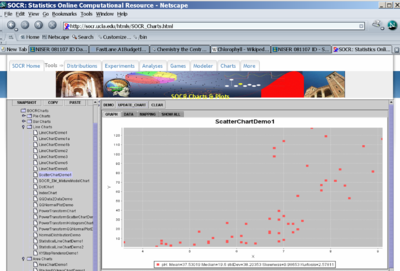
- Regression Plots (Relations between Calcium, Chlorophyll and Avg_Mercury)
- TBD
Model Fitting
- Model 1
- Model goodness-of-fit assessment
Examples
Computer simulations vs. real observed data
Hands-on activities
Step-by-step practice problems.
Notes
- Follow the directions in the SOCR Wiki Editing Guide to expand, revise or improve these materials.
Translate this page: