Difference between revisions of "SMHS SciVisualization SOCR Excel R Charts"
(→See Next) |
(→Heatmaps) |
||
(9 intermediate revisions by one other user not shown) | |||
Line 3: | Line 3: | ||
==SOCR Charts== | ==SOCR Charts== | ||
− | + | *URL: http://socr.umich.edu/html/cha/ (Java applet) | |
− | + | *About/List: http://wiki.stat.ucla.edu/socr/index.php/About_pages_for_SOCR_Chart_List | |
− | + | *Activities: http://wiki.stat.ucla.edu/socr/index.php/SOCR_EduMaterials_ChartsActivities | |
− | + | *Data: http://wiki.socr.umich.edu/index.php/SOCR_Data | |
<center>[[Image:SMHS_SciVisualization2.png|500px]] </center> | <center>[[Image:SMHS_SciVisualization2.png|500px]] </center> | ||
Line 135: | Line 135: | ||
===Examples of powerful R interactive visualization packages=== | ===Examples of powerful R interactive visualization packages=== | ||
− | + | *<b>ggplot2</b> – http://ggplot2.org | |
− | + | *<b>ggvis</b> – interactive plots extending the static ggplot2 charts, http://ggvis.rstudio.com | |
− | + | *<b>rCharts</b> – R interface to multiple JavaScript charting libraries, http://rcharts.io | |
− | + | *<b>plotly</b> – transforming ggplot2 charts into interactive plots, https://plot.ly/r | |
− | + | *<b>googleVis</b> – Google Charts using R, http://cran.r-project.org/web/packages/googleVis/vignettes/googleVis_examples.html | |
− | + | *<b>HTMLWidgets</b> | |
− | + | ** <b>leaflet</b> – library for creating dynamic maps, supports panning and zooming, annotations, markers, polygons, etc. http://www.htmlwidgets.org/showcase_leaflet.html | |
+ | ** <b>dygraphs</b> – provides mechanism for charting time-series data, supports interactive navigation features including series/point highlighting, zooming, and panning, http://www.htmlwidgets.org/showcase_dygraphs.html | ||
+ | ** <b>networkD3</b> – library for creating D3 network graphs including force directed networks, Sankey diagrams, and Reingold-Tilford tree networks, http://www.htmlwidgets.org/showcase_networkD3.html | ||
+ | ** <b>DataTables</b> – displays R matrices or data frames as interactive HTML tables that support filtering, pagination, and sorting, http://www.htmlwidgets.org/showcase_datatables.html | ||
+ | ** <b>Rthreejs</b> – features 3D scatterplots and globes based on WebGL, http://www.htmlwidgets.org/showcase_threejs.html | ||
− | + | *Other R graphic examples | |
− | + | ** To write out plots out to file use: | |
− | |||
− | |||
− | |||
− | |||
− | |||
− | |||
− | |||
− | |||
# pdf() command all graphs are redirected to test.pdf. Also works with other common formats: jpeg, png, ps, tiff. | # pdf() command all graphs are redirected to test.pdf. Also works with other common formats: jpeg, png, ps, tiff. | ||
pdf("C:\\Users\\Dinov\\Desktop\\test.pdf"); plot(1:100, 1:100); dev.off() | pdf("C:\\Users\\Dinov\\Desktop\\test.pdf"); plot(1:100, 1:100); dev.off() | ||
Line 405: | Line 401: | ||
===Heatmaps=== | ===Heatmaps=== | ||
− | # Generating Dendogram Association Heatmap Plot (Genotype vs. Imaging phenotype | + | # Generating Dendogram Association Heatmap Plot (Genotype vs. Imaging phenotype) |
# http://stat.ethz.ch/R-manual/R-patched/library/stats/html/heatmap.html | # http://stat.ethz.ch/R-manual/R-patched/library/stats/html/heatmap.html | ||
# http://www.ncbi.nlm.nih.gov/pmc/articles/PMC4005931/ | # http://www.ncbi.nlm.nih.gov/pmc/articles/PMC4005931/ | ||
Line 439: | Line 435: | ||
<center>[[Image:SMHS_SciVisualization26.png|500px]] </center> | <center>[[Image:SMHS_SciVisualization26.png|500px]] </center> | ||
− | # Alternatively, we can use the R package gplots | + | # Alternatively, we can use the R package '''gplots''' |
install.packages("gplots") | install.packages("gplots") | ||
library(gplots) | library(gplots) | ||
Line 451: | Line 447: | ||
===Correlation Plots=== | ===Correlation Plots=== | ||
− | The <b>corrplot</b> package is a graphical display of a correlation matrix | + | The <b>corrplot</b> package is a graphical display of a correlation matrix and confidence intervals, with some tools for matrix reordering. There are seven visualization methods (parameter method) in corrplot package, named "circle", "square", "ellipse", "number", "shade", "color", "pie". |
# install.packages("corrplot") | # install.packages("corrplot") | ||
library(corrplot) | library(corrplot) | ||
Line 461: | Line 457: | ||
===Hyperbolic Visualization=== | ===Hyperbolic Visualization=== | ||
− | + | *URL: http://socr.umich.edu/html/Navigators.html | |
− | |||
− | |||
− | |||
− | |||
− | |||
− | |||
− | |||
− | |||
− | |||
− | |||
− | |||
− | |||
− | + | *Tools: | |
+ | ** Java/Jar applet: http://socr.umich.edu/html/navigators/HW/jars/SOCR_HW_Viewer.jar | ||
+ | ** JavaScript: http://socr.umich.edu/html/navigators/D3/SOCR_D3_Viewer.html (JSON) | ||
− | + | *Data Format | |
+ | ** XML data: http://socr.umich.edu/html/navigators/HW/SOCR_HyperTree.xml | ||
+ | ** JSON data: http://socr.umich.edu/html/navigators/D3/xml/SOCR_HyperTree.json | ||
− | + | *D3 Visualization | |
+ | ** E:\Ivo.dir\Research\UMichigan\Education_Teaching_Curricula\2015_2016\HS_853_Fall_2015\Modules_docx\Tools\TreeViewer_JS | ||
+ | ** treeJS.json | ||
+ | ** flareD3.json | ||
− | < | + | <center>[[Image:SMHS_SciVisualization29.png|400px]] </center> |
− | <center>[[Image: | + | <center>[[Image:SMHS_SciVisualization30.png|400px]] </center> |
− | + | *URL: https://github.com/mbostock/d3/wiki/Gallery | |
− | + | *Source code: https://github.com/mbostock/d3 | |
− | + | <center>[[Image:SMHS_SciVisualization31.png|400px]] </center> | |
− | |||
− | <center>[[Image:SMHS_SciVisualization31.png| | ||
===Motion Charts=== | ===Motion Charts=== | ||
− | + | *Video: http://www.socr.ucla.edu/SOCR_MotionCharts/SOCR_HTML5_MotionChart_Video2.gif | |
− | + | *Java: http://www.socr.ucla.edu/SOCR_MotionCharts/ | |
− | + | *HTML5: http://socr.umich.edu/HTML5/MotionChart/ | |
− | + | *Activities: http://wiki.socr.umich.edu/index.php/SOCR_MotionCharts | |
Line 508: | Line 497: | ||
===1D/2D/3D signal/area/volume/surface/model/atlas visualization=== | ===1D/2D/3D signal/area/volume/surface/model/atlas visualization=== | ||
− | + | *1D: (See R/SOCR Visualization tools above) | |
− | + | *2D: http://imagej.nih.gov/ij/ | |
− | + | *3D: http://socr.umich.edu/HTML5/BrainViewer/ | |
<b>Supported File Formats:</b> | <b>Supported File Formats:</b> | ||
Line 523: | Line 512: | ||
===Trees and Graphs=== | ===Trees and Graphs=== | ||
− | + | *Trees/Hierarchies and general Graphs | |
# Install and load the APE package, needed for the phylogenetic tree rendering (as.phylo) | # Install and load the APE package, needed for the phylogenetic tree rendering (as.phylo) | ||
Line 575: | Line 564: | ||
You can now cut the tree into branches. You can split the tree into 2 groups, by setting the number of cuts with the k=2 parameter, or by specifying height to cut the tree at (?cutree): | You can now cut the tree into branches. You can split the tree into 2 groups, by setting the number of cuts with the k=2 parameter, or by specifying height to cut the tree at (?cutree): | ||
− | + | k- an integer scalar or vector with the desired number of groups | |
− | + | ||
+ | h- numeric scalar or vector with heights where the tree should be cut | ||
cutree(hc, k = 2) | cutree(hc, k = 2) | ||
Line 650: | Line 640: | ||
===Publications=== | ===Publications=== | ||
− | + | *This paper examines nasal and bronchial tissue cultures as appropriate in vitro models for the assessment of smoking-induced adverse effects in the respiratory system (doi: 10.1177/1091581814551647), using “hclust” package. No data. | |
− | + | *This paper classified subtypes of gastric cancer based on epidemiologic and histologic and gene expression data. These new classifications of gastric cancer have implications for improving our understanding of disease biology and identification of unique molecular drivers for each gastric cancer subtype (doi: 10.1158/1078-0432.CCR-10-2203). | |
<b>Repeat the clustering</b> | <b>Repeat the clustering</b> | ||
Line 781: | Line 771: | ||
==See Next== | ==See Next== | ||
− | + | *[[SMHS_SciVisualization_NetworkViz|Next See the Complex Network Visualization section]] | |
− | + | *[[SMHS| Back to the Scientific Methods for Health Sciences EBook ToC]] | |
<hr> | <hr> | ||
* SOCR Home page: http://www.socr.umich.edu | * SOCR Home page: http://www.socr.umich.edu | ||
{{translate|pageName=http://wiki.socr.umich.edu/index.php?title=SMHS_SciVisualization_SOCR_Excel_R_Charts}} | {{translate|pageName=http://wiki.socr.umich.edu/index.php?title=SMHS_SciVisualization_SOCR_Excel_R_Charts}} |
Latest revision as of 12:53, 23 May 2016
Contents
SMHS Scientific Visualization - SOCR, Excel and R charts
SOCR Charts
- URL: http://socr.umich.edu/html/cha/ (Java applet)
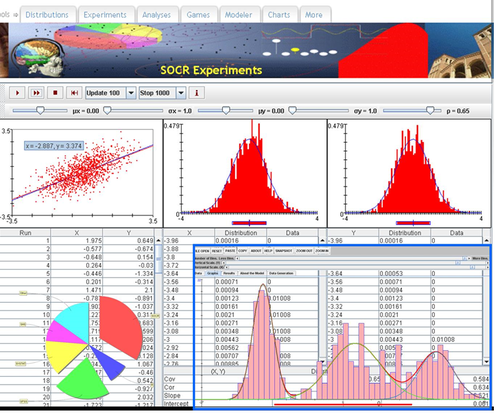
Excel Charts
MS Excel provides a large number of charts that can be used to quickly and effectively render complex multivariate data. For instance, the table below contains the principal component analysis (PCA) of 50 derived neuroimaging biomarkers (region of interest (ROI) by shape morphometry metric). The loadings of these 50 variables on the top 5 (most significant) principal component directions are shown in the table. Experiment with effective visualizations of these data.
Hemi | ROI | measure | ROI_Measure | Dim.1 | Dim.2 | Dim.3 | Dim.4 | Dim.5 |
---|---|---|---|---|---|---|---|---|
L | insular | AvgMeanCurvature | L_insular_cortex_AvgMeanCurvature | 0.72 | 0 | 0.06 | 0.06 | 0 |
L | insular | ComputeArea | L_insular_cortex_ComputeArea | 0.77 | 0.06 | 0.04 | 0.01 | 0 |
L | insular | Volume | L_insular_cortex_Volume | 0.72 | 0.09 | 0.04 | 0.03 | 0.01 |
L | insular | ShapeIndex | L_insular_cortex_ShapeIndex | 0.46 | 0.06 | 0.01 | 0.02 | 0.01 |
L | insular | Curvedness | L_insular_cortex_Curvedness | 0.78 | 0 | 0.05 | 0.03 | 0.01 |
R | insular | AvgMeanCurvature | R_insular_cortex_AvgMeanCurvature | 0.79 | 0 | 0.03 | 0.08 | 0 |
R | insular | ComputeArea | R_insular_cortex_ComputeArea | 0.79 | 0.09 | 0.03 | 0.01 | 0 |
R | insular | Volume | R_insular_cortex_Volume | 0.73 | 0.11 | 0.03 | 0.03 | 0 |
R | insular | ShapeIndex | R_insular_cortex_ShapeIndex | 0.27 | 0.17 | 0 | 0.07 | 0 |
R | insular | Curvedness | R_insular_cortex_Curvedness | 0.84 | 0.02 | 0.03 | 0.01 | 0 |
L | cingulate | AvgMeanCurvature | L_cingulate_gyrus_AvgMeanCurvature | 0.72 | 0 | 0.05 | 0.02 | 0.02 |
L | cingulate | ComputeArea | L_cingulate_gyrus_ComputeArea | 0.74 | 0.05 | 0.06 | 0.04 | 0.01 |
L | cingulate | Volume | L_cingulate_gyrus_Volume | 0.69 | 0.08 | 0.05 | 0.05 | 0.01 |
L | cingulate | ShapeIndex | L_cingulate_gyrus_ShapeIndex | 0.53 | 0 | 0.05 | 0 | 0.03 |
L | cingulate | Curvedness | L_cingulate_gyrus_Curvedness | 0.7 | 0.01 | 0.05 | 0.04 | 0.03 |
R | cingulate | AvgMeanCurvature | R_cingulate_gyrus_AvgMeanCurvature | 0.6 | 0 | 0.02 | 0.03 | 0.01 |
R | cingulate | ComputeArea | R_cingulate_gyrus_ComputeArea | 0.73 | 0.06 | 0.04 | 0.03 | 0.01 |
R | cingulate | Volume | R_cingulate_gyrus_Volume | 0.68 | 0.09 | 0.04 | 0.04 | 0.01 |
R | cingulate | ShapeIndex | R_cingulate_gyrus_ShapeIndex | 0.56 | 0.01 | 0.05 | 0 | 0.01 |
R | cingulate | Curvedness | R_cingulate_gyrus_Curvedness | 0.25 | 0 | 0.01 | 0.04 | 0 |
L | caudate | AvgMeanCurvature | L_caudate_AvgMeanCurvature | 0.52 | 0 | 0.05 | 0 | 0.01 |
L | caudate | ComputeArea | L_caudate_ComputeArea | 0.51 | 0.09 | 0.03 | 0.04 | 0.02 |
L | caudate | Volume | L_caudate_Volume | 0.44 | 0.09 | 0.03 | 0.06 | 0.03 |
L | caudate | ShapeIndex | L_caudate_ShapeIndex | 0.2 | 0.03 | 0.04 | 0.04 | 0 |
L | caudate | Curvedness | L_caudate_Curvedness | 0.51 | 0.12 | 0.02 | 0.01 | 0.01 |
R | caudate | AvgMeanCurvature | R_caudate_AvgMeanCurvature | 0.68 | 0.04 | 0.04 | 0.02 | 0 |
R | caudate | ComputeArea | R_caudate_ComputeArea | 0.67 | 0.17 | 0.03 | 0.02 | 0.01 |
R | caudate | Volume | R_caudate_Volume | 0.61 | 0.16 | 0.02 | 0.03 | 0.01 |
R | caudate | ShapeIndex | R_caudate_ShapeIndex | 0.18 | 0.02 | 0.03 | 0.11 | 0 |
R | caudate | Curvedness | R_caudate_Curvedness | 0.65 | 0.19 | 0.01 | 0 | 0 |
L | putamen | AvgMeanCurvature | L_putamen_AvgMeanCurvature | 0.62 | 0 | 0.04 | 0.03 | 0.02 |
L | putamen | ComputeArea | L_putamen_ComputeArea | 0.56 | 0.05 | 0.04 | 0.03 | 0.05 |
L | putamen | Volume | L_putamen_Volume | 0.52 | 0.07 | 0.04 | 0.05 | 0.05 |
L | putamen | ShapeIndex | L_putamen_ShapeIndex | 0.06 | 0.13 | 0 | 0.15 | 0 |
L | putamen | Curvedness | L_putamen_Curvedness | 0.64 | 0.11 | 0.03 | 0.01 | 0.03 |
R | putamen | AvgMeanCurvature | R_putamen_AvgMeanCurvature | 0.62 | 0 | 0.07 | 0.04 | 0.01 |
R | putamen | ComputeArea | R_putamen_ComputeArea | 0.66 | 0.08 | 0.03 | 0.01 | 0.03 |
R | putamen | Volume | R_putamen_Volume | 0.64 | 0.12 | 0.03 | 0.02 | 0.03 |
R | putamen | ShapeIndex | R_putamen_ShapeIndex | 0.15 | 0.24 | 0 | 0.08 | 0.03 |
R | putamen | Curvedness | R_putamen_Curvedness | 0.65 | 0.05 | 0.05 | 0 | 0.02 |
L | hippocampus | AvgMeanCurvature | L_hippocampus_AvgMeanCurvature | 0.78 | 0 | 0.01 | 0.04 | 0 |
L | hippocampus | ComputeArea | L_hippocampus_ComputeArea | 0.75 | 0.07 | 0.01 | 0 | 0.02 |
L | hippocampus | Volume | L_hippocampus_Volume | 0.72 | 0.09 | 0.01 | 0.01 | 0.01 |
L | hippocampus | ShapeIndex | L_hippocampus_ShapeIndex | 0.45 | 0.17 | 0 | 0.04 | 0.02 |
L | hippocampus | Curvedness | L_hippocampus_Curvedness | 0.79 | 0.03 | 0.01 | 0 | 0.02 |
R | hippocampus | AvgMeanCurvature | R_hippocampus_AvgMeanCurvature | 0.72 | 0 | 0 | 0.1 | 0.01 |
R | hippocampus | ComputeArea | R_hippocampus_ComputeArea | 0.71 | 0.09 | 0 | 0 | 0.05 |
R | hippocampus | Volume | R_hippocampus_Volume | 0.68 | 0.1 | 0 | 0 | 0.04 |
R | hippocampus | ShapeIndex | R_hippocampus_ShapeIndex | 0.37 | 0.18 | 0 | 0.02 | 0.03 |
R | hippocampus | Curvedness | R_hippocampus_Curvedness | 0.77 | 0.03 | 0 | 0.02 | 0.04 |
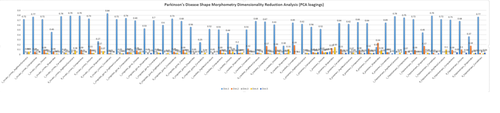
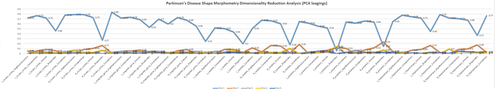

R-Charts
There are 100’s of packages and 1,000 of different charts, plots and graphs that can be generated using R. Such interactive visualizations enable deeper exploration of data, models and results. JavaScript libraries, e.g., D3, provide advantages for data visualization as these involve HTML5 and are easily shareable online. The R community is developing R interfaces to some popular JavaScript libraries to allow users to create interactive visualizations without detailed knowledge of JavaScript.
Examples of powerful R interactive visualization packages
- ggplot2 – http://ggplot2.org
- ggvis – interactive plots extending the static ggplot2 charts, http://ggvis.rstudio.com
- rCharts – R interface to multiple JavaScript charting libraries, http://rcharts.io
- plotly – transforming ggplot2 charts into interactive plots, https://plot.ly/r
- googleVis – Google Charts using R, http://cran.r-project.org/web/packages/googleVis/vignettes/googleVis_examples.html
- HTMLWidgets
- leaflet – library for creating dynamic maps, supports panning and zooming, annotations, markers, polygons, etc. http://www.htmlwidgets.org/showcase_leaflet.html
- dygraphs – provides mechanism for charting time-series data, supports interactive navigation features including series/point highlighting, zooming, and panning, http://www.htmlwidgets.org/showcase_dygraphs.html
- networkD3 – library for creating D3 network graphs including force directed networks, Sankey diagrams, and Reingold-Tilford tree networks, http://www.htmlwidgets.org/showcase_networkD3.html
- DataTables – displays R matrices or data frames as interactive HTML tables that support filtering, pagination, and sorting, http://www.htmlwidgets.org/showcase_datatables.html
- Rthreejs – features 3D scatterplots and globes based on WebGL, http://www.htmlwidgets.org/showcase_threejs.html
- Other R graphic examples
- To write out plots out to file use:
# pdf() command all graphs are redirected to test.pdf. Also works with other common formats: jpeg, png, ps, tiff. pdf("C:\\Users\\Dinov\\Desktop\\test.pdf"); plot(1:100, 1:100); dev.off() # Generates Scalable Vector Graphics (SVG) that can be edited by vector graphics software svg("test.svg"); plot(1:100, 1:100); dev.off()
Paired ScatterPlots
set.seed(100) x <- matrix(runif(50), ncol=5, dimnames=list(letters[1:10], LETTERS[1:5])) describe(x) # library("Hmisc") plot(x[,1], x[,2], pch=20, col="red", main="Symbols and Labels") text(x[,1]+0.03, x[,2], rownames(x))

pairs(x)

Another way to generate scatterplots is by using ggplot:
# library(ggplot2) x <- sample(1:20, 20); y <- sample(1:20, 20); cat <- rep(c("A", "B", "C", "D"), 5) #vs. cat <- rep(c("A", "B", "C", "D"), each=5) plot.1 <- qplot(x, y, geom="point", size=5*x, color=cat, main="GGplot with Relative Dot Size and Color") + theme(legend.position = "topleft") print(plot.1)
# Use Case-Studies: https://umich.instructure.com/courses/38100/files/folder/Case_Studies # Case_03_MentalHealthServicesSurvey # data1 <- read.table('https://umich.instructure.com/files/399128/download?download_frd=1&verifier=AG2e9QUKUm1jvDBpkX7D9jbEjKNc4irA0ECk0f7p', header=T) head(data1) attach(data1) # library("Hmisc") describe(data1)
plot(data1[,3], data1[,4], pch=20, col="red", main="Symbols and Labels") # text(data1 [,3]+0.03, data1 [,4], rownames(data1)) plot.1 <- qplot(x, y, geom="point", size=5*x, color=cat, main="GGplot with Relative Dot Size and Color") + theme(legend.position = "topleft") print(plot.1)
# redo plots using majorfundtype FacilityType Ownership Focus # pairs(data1, na.action=na.omit)

# Scatterplot with regression line. Use the “diamonds” dataset, which is a data frame with # 53,940 rows and 10 variables () # describe(diamonds)
# Use Case-Studies: https://umich.instructure.com/courses/38100/files/folder/Case_Studies # CaseStudy01_Divorce_YoungAdults # data1 <- read.csv('https://umich.instructure.com/files/399118/download?download_frd=1&verifier=ESACv31KcyiHbkPZPuT8Oo4V7XzPtgTTbs6PQLTv', header=T) attach(data1) # plot variables: DIVYEAR momint dadint momclose depression livewithmom gethitched
set.seed(110) # par(mfrow=c(1,2)) data.2 <- diamonds[sample(nrow(diamonds), 500), ] plot.2 <- qplot(price, depth, data = data.2, geom = c("point", "smooth"), method = "lm") plot.3 <- qplot(carat, price, data=data.2, geom=c("point", "smooth"), span=0.4) print(plot.2); print(plot.3)
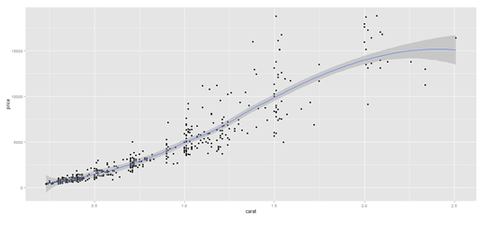
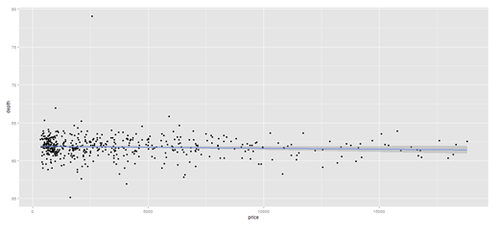
Barplots
x <- matrix(runif(50), ncol=5, dimnames=list(letters[1:10], LETTERS[1:5])) barplot(x[1:4,], ylim=c(0, max(x[1:4,])+0.3), beside=TRUE, legend.text = letters[1:4], args.legend = list(x = "topleft")) text(labels=round(as.vector(as.matrix(x[1:4,])),2), x=seq(1.5, 21, by=1) + sort(rep(c(0,1,2,3,4), 4)), y=as.vector(as.matrix(x[1:4,]))+0.1)

# to put error bars on barplot:
# 10 rows (a, b, c, …): bar <- barplot(m <- rowMeans(x) * 10, ylim=c(0, 10)) stdev <- sd(t(x)) arrows(bar, m, bar, m + stdev, length=0.15, angle = 90)
# Case_04_ChildTrauma # data1 <- read.table('https://umich.instructure.com/files/399129/download?download_frd=1&verifier=Hmv0YW2Kie5ZTV9CKBUNArSHR66f3GWSmVzZDBxc', header=T) attach(data1) head(x) head(data1) # plot data data2 <- data1[,-5] # remove the 5th columns text data1 <- data2[,-5] # remove the 6th columns text # or data1 <- data1[,c(-5,-6)]
data2 <- as.data.frame(data1) Blacks <- data2[which(data2$\$$race=="black"),] Other <- data2[which(data2$\$$race=="other"),] Hispanic <- data2[which(data2$\$$race=="hispanic"),] White <- data2[which(data2$\$$race=="white"),]
A <- c(mean(Blacks$\$$age), mean(Blacks$\$$service)) #colnames(A) <- c("age "," service ") B <- c(mean(Other$\$$age), mean(Other$\$$service)) C <- c(mean(Hispanic$\$$age), mean(Hispanic$\$$service)) D <- c(mean(White$\$$age), mean(White$\$$service))
x <- cbind(A, B, C, D)
bar <- barplot(x[1:2,], ylim=c(0, max(x[1:2,])+2.0), beside=TRUE, legend.text = c("age","service") , args.legend = list(x = "right")) text(labels=round(as.vector(as.matrix(x[1:2,])),2), x=seq(1.4, 21, by=1.5), #y=as.vector(as.matrix(x[1:2,]))+0.3)
y=11.5)
m <- x; stdev <- sd(t(x)) arrows(bar, m, bar, m + stdev, length=0.15, angle = 90)
barplot(as.matrix(data1[1:4,]), ylim=c(0, max(data1[1:4,])+0.3), beside=TRUE, legend.text = data1[1:4,1], args.legend = list(x = "topleft")) text(labels=round(as.vector(as.matrix(data1[1:4,])),2), x=seq(1.5, 21, by=1), y=as.vector(as.matrix(data1[1:4,]))+0.1)

# Columns (A, B, C, D, E): bar <- barplot(m <- colMeans(x) * 5, ylim=c(0, 5)) stdev <- sd(t(x)) arrows(bar, m, bar, m + stdev, length=0.15, angle = 90)

Histograms and Density Plots
hist(x, freq=TRUE, breaks=10)
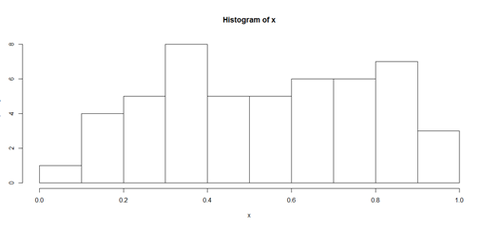
plot(density(x), lwd = 10, col="green")
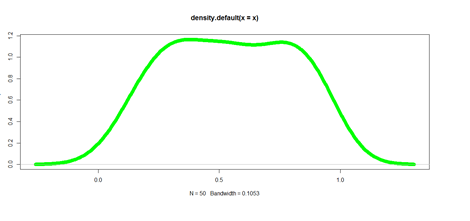
Pie Chart
# first , “A”, and second, “B”, columns par (mfrow=c(1,2)) pie(x[,1], col=rainbow(length(x[,1]), start=0.1, end=0.8), clockwise=TRUE)
pie(x[,1], col=rainbow(length(x[,1]), start=0.1, end=0.8), clockwise=TRUE)
pie(x[,2], col=rainbow(length(x[,2]), start=0.1, end=0.8), clockwise=TRUE) legend("topleft", legend=row.names(x), cex=1.3, bty="n", pch=15, pt.cex=1.8, col=rainbow(length(x[,2]), start=0.1, end=0.8), ncol=1)
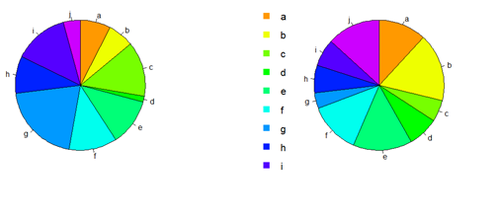
You can export the data: write.table(x, " ", "data.txt") # copy-paste it in SOCR Pie chart to generate another Pie view of data
Line Plots Using ggplot
head(diamonds)
Carat | Cut | Color | Clarity | Depth | Table | Price | X | Y | Z | |
1 | 0.23 | Ideal | E | SI2 | 61.5 | 55 | 326 | 3.95 | 3.98 | 2.43 |
2 | 0.21 | Premium | E | SI1 | 59.8 | 61 | 326 | 3.89 | 3.84 | 2.31 |
3 | 0.23 | Good | E | VS1 | 56.9 | 65 | 237 | 4.05 | 4.07 | 2.31 |
4 | 0.29 | Premium | I | VS2 | 62.4 | 58 | 334 | 4.2 | 4.23 | 2.63 |
5 | 0.31 | Good | J | SI2 | 63.3 | 58 | 335 | 4.34 | 4.35 | 4.75 |
6 | 0.24 | VeryGood | J | VVS2 | 62.8 | 57 | 336 | 3.94 | 3.96 | 2.48 |
plot.2 <- ggplot(diamonds, aes(carat, price, group=cut, color=cut)) + geom_line() print(plot.2)
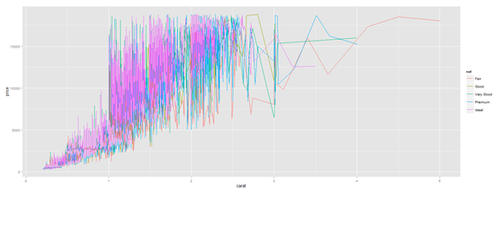
plot.2 <- ggplot(data1, aes(age, service, group=race, color=race)) + geom_line() print(plot.2)
# Faceting plot (geometrically, faceting (or facetting) is the process of removing parts of a polygon, polyhedron or polytope, without creating any new vertices) plot.3 <- ggplot(diamonds, aes(carat, price)) + geom_line(aes(color=cut), size=1) + facet_wrap(~cut, ncol=1) print(plot.3)

Barplots with ggplot
plot.4 <- ggplot(diamonds, aes(cut, fill=cut)) + geom_bar() + facet_grid(. ~ clarity) print(plot.4)

New_var <- service+rnorm(1000, 0,1) data1$\$$New_var <- int(New_var) plot.4 <- ggplot(data1, aes(race, fill= traumatype)) + geom_bar() + facet_grid(. ~ New_var) print(plot.4) plot.4a <- ggplot(diamonds, aes(color, price/carat, fill=color)) + geom_boxplot() print(plot.4a) <center>[[Image:SMHS_SciVisualization20.png|500px]] </center> ==='"`UNIQ--h-11--QINU`"'Jitter plot=== plot.5 <- ggplot(diamonds, aes(color, price/carat)) + geom_jitter(alpha = I(1 / 2), aes(color=color)) print(plot.5) <center>[[Image:SMHS_SciVisualization21.png|500px]] </center> ==='"`UNIQ--h-12--QINU`"'Density Plots=== plot.6 <- ggplot(diamonds, aes(carat, size=2)) + geom_density(aes(color = cut)) print(plot.6) <center>[[Image:SMHS_SciVisualization22.png|500px]] </center> plot.6 <- ggplot(data1, aes(age, size=2)) + geom_density(aes(color = traumatype)) print(plot.6) plot.7 <- ggplot(diamonds, aes(carat, size=2)) + geom_density(aes(fill = color)) print(plot.7) <center>[[Image:SMHS_SciVisualization23.png|500px]] </center> plot.8 <- ggplot(diamonds, aes(x=carat, size=1)) + geom_histogram(aes(y = price), binwidth=0.2) + geom_density() print(plot.8) <center>[[Image:SMHS_SciVisualization24.png|500px]] </center> plot.8a <- ggplot(diamonds, aes(x=carat, size=1)) + geom_histogram(aes(y = price), stat="identity") + geom_density() print(plot.8a) <center>[[Image:SMHS_SciVisualization25.png|500px]] </center> ==='"`UNIQ--h-13--QINU`"'Heatmaps=== # Generating Dendogram Association Heatmap Plot (Genotype vs. Imaging phenotype) # http://stat.ethz.ch/R-manual/R-patched/library/stats/html/heatmap.html # http://www.ncbi.nlm.nih.gov/pmc/articles/PMC4005931/ AD_Associations_Data <- read.table("https://umich.instructure.com/files/330387/download?download_frd=1&verifier=gLk2ADgrLhXGeknI6mqIeJugi2ODr8RARsQlBUMe", header=TRUE, row.names=1, sep=",", dec=".") MCI_Associations_Data <- read.table("https://umich.instructure.com/files/330390/download?download_frd=1&verifier=FczlJD6ISRPZhu69xvHuoZHx2c7gXX9YEvvPCTBG", header=TRUE, row.names=1, sep=",", dec=".") NC_Associations_Data <- read.table("https://umich.instructure.com/files/330391/download?download_frd=1&verifier=i2BEtSpmpbrzQUPoA2ST06IzzcaenyVEHRepHSF3", header=TRUE, row.names=1, sep=",", dec=".") require(graphics) require(grDevices) library(gplots) AD_Data <- AD_Associations_Data MCI_Data <- MCI_Associations_Data NC_Data <- NC_Associations_Data AD_mat <- as.matrix(AD_Data); class(AD_mat) <- "numeric" MCI_mat <- as.matrix(MCI_Data); class(MCI_mat) <- "numeric" NC_mat <- as.matrix(NC_Data); class(NC_mat) <- "numeric" # set up the rol (rc) and column (cc) colors for each cohort rcAD <- rainbow(nrow(AD_mat), start = 0, end = 1.0); ccAD<-rainbow(ncol(AD_mat), start = 0, end = 1.0) rcMCI <- rainbow(nrow(MCI_mat), start = 0, end=1.0); ccMCI<-rainbow(ncol(MCI_mat),start=0,end=1.0) rcNC <- rainbow(nrow(NC_mat), start = 0, end = 1.0); ccNC<-rainbow(ncol(NC_mat), start = 0, end = 1.0) # set up 1x3 graph display - par (mfrow=c(1,3)) – does not work with ‘heatmap’ hvAD <- heatmap(AD_mat, col = cm.colors(256), scale = "column", RowSideColors = rcAD, ColSideColors = ccAD, margins = c(2,2), main="AD Cohort SNP-ROI_volume Association (p_values)") hvMCI <- heatmap(MCI_mat, col = cm.colors(256), scale = "column", RowSideColors = rcMCI, ColSideColors = ccMCI, margins = c(2,2), main="MCI Cohort SNP-ROI_volume Association (p_values)") hvNC <- heatmap(NC_mat, col = cm.colors(256), scale = "column", RowSideColors = rcNC, ColSideColors = ccNC, margins = c(2,2), main="NC Cohort SNP-ROI_volume Association (p_values)") <center>[[Image:SMHS_SciVisualization26.png|500px]] </center> # Alternatively, we can use the R package '''gplots''' install.packages("gplots") library(gplots) ## col dendrogram plotted and col reordering done. # heatmap.2(AD_mat, keysize=2) ## A more decorative heatmap, with z-score scaling along columns heatmap.2(AD_mat, col=cm.colors(255), scale="column", RowSideColors=rcAD, ColSideColors=ccAD, margin=c(8, 7), xlab="Imaging Biomarkers (ROI volume)", ylab= "Genetics Biomarkers (SNPs)", main="AD Associations Heatmap (SNP-Imaging)", tracecol="green", density="density") <center>[[Image:SMHS_SciVisualization27.png|500px]] </center> ==='"`UNIQ--h-14--QINU`"'Correlation Plots=== The <b>corrplot</b> package is a graphical display of a correlation matrix and confidence intervals, with some tools for matrix reordering. There are seven visualization methods (parameter method) in corrplot package, named "circle", "square", "ellipse", "number", "shade", "color", "pie". # install.packages("corrplot") library(corrplot) NC_Associations_Data <- read.table("https://umich.instructure.com/files/330391/download?download_frd=1&verifier=i2BEtSpmpbrzQUPoA2ST06IzzcaenyVEHRepHSF3", header=TRUE, row.names=1, sep=",", dec=".") M <- cor(NC_Associations_Data) <center>[[Image:SMHS_SciVisualization28.png|500px]] </center> ==='"`UNIQ--h-15--QINU`"'Hyperbolic Visualization=== *URL: http://socr.umich.edu/html/Navigators.html *Tools: ** Java/Jar applet: http://socr.umich.edu/html/navigators/HW/jars/SOCR_HW_Viewer.jar ** JavaScript: http://socr.umich.edu/html/navigators/D3/SOCR_D3_Viewer.html (JSON) *Data Format ** XML data: http://socr.umich.edu/html/navigators/HW/SOCR_HyperTree.xml ** JSON data: http://socr.umich.edu/html/navigators/D3/xml/SOCR_HyperTree.json *D3 Visualization ** E:\Ivo.dir\Research\UMichigan\Education_Teaching_Curricula\2015_2016\HS_853_Fall_2015\Modules_docx\Tools\TreeViewer_JS ** treeJS.json ** flareD3.json <center>[[Image:SMHS_SciVisualization29.png|400px]] </center> <center>[[Image:SMHS_SciVisualization30.png|400px]] </center> *URL: https://github.com/mbostock/d3/wiki/Gallery *Source code: https://github.com/mbostock/d3 <center>[[Image:SMHS_SciVisualization31.png|400px]] </center> ==='"`UNIQ--h-16--QINU`"'Motion Charts=== *Video: http://www.socr.ucla.edu/SOCR_MotionCharts/SOCR_HTML5_MotionChart_Video2.gif *Java: http://www.socr.ucla.edu/SOCR_MotionCharts/ *HTML5: http://socr.umich.edu/HTML5/MotionChart/ *Activities: http://wiki.socr.umich.edu/index.php/SOCR_MotionCharts <center>[[Image:SMHS_SciVisualization32.png|500px]] </center> ==='"`UNIQ--h-17--QINU`"'1D/2D/3D signal/area/volume/surface/model/atlas visualization=== *1D: (See R/SOCR Visualization tools above) *2D: http://imagej.nih.gov/ij/ *3D: http://socr.umich.edu/HTML5/BrainViewer/ <b>Supported File Formats:</b> Volumes (.nii / .nii.gz / .img&.hdr / .mgh / .mgz / .nrrd) Shapes (.dx / .vtk / .stl / FreeSurfer) Fibers (.trk) <center>[[Image:SMHS_SciVisualization33.png|500px]] </center> ==='"`UNIQ--h-18--QINU`"'Trees and Graphs=== *Trees/Hierarchies and general Graphs # Install and load the APE package, needed for the phylogenetic tree rendering (as.phylo) # install.packages("ape") library("ape") Load data # Data: 02_Nof1_Data.csv data.1 <- read.table("https://umich.instructure.com/files/330385/download?download_frd=1&verifier=DwJUGSd6t24dvK7uYmzA2aDyzlmsohyaK6P7jK0Q ", sep=",", header = TRUE) head(data.1) <center> {| class="wikitable" style="text-align:center; width:35%" border="1" |- mydata1 |- |||ID||Day||Tx||SelfEff||SelfEff25||WPSS||SocSuppt||PMss||PMss3||PhyAct |- |1||1||1||1||33||8||0.97||5.00||4.03||1.03||53 |- |2||1||2||1||33||8||-0.17||3.87||4.03||1.03||73 |- |3||1||3||0||33||8||0.81||4.84||4.03||1.03||23 |- |4||1||4||0||33||8||-0.41||3.62||4.03||1.03||36 |- |5||1||5||1||33||8||0.59||4.62||4.03||1.03||21 |- |6||1||6||1||33||8||-1.16||2.87||4.03||1.03||0 |} </center> Clustering hc = hclust(dist(data.1), 'ave') # the agglomeration method can be specified "ward.D", "ward.D2", "single","complete", "average" (= UPGMA), "mcquitty" (= WPGMA),"median" (= WPGMC) or "centroid" (= UPGMC) # (3) Plot clustering diagram par (mfrow=c(1,1)) # very simple dendrogram plot(hc) <center>[[Image:SMHS_SciVisualization34.png|500px]] </center> require(graphics) (x <- identify(hc)) ## Terminate with 2nd mouse button !! identify(hc, <mark>function(k)</mark> print(table(data.1[k,5]))) You can now cut the tree into branches. You can split the tree into 2 groups, by setting the number of cuts with the k=2 parameter, or by specifying height to cut the tree at (?cutree): k- an integer scalar or vector with the desired number of groups h- numeric scalar or vector with heights where the tree should be cut cutree(hc, k = 2) # alternatively specify the height, which is, the value of the criterion associated with the clustering method # for the particular agglomeration. cutree(hc, h= 50) # cut at h=50 table(cutree(hc, h= 50)) # cluster distribution # To identify the number of cases for varying number of clusters we can combine calls to cutree and table # in a call to <b>sapply</b> -- to see the sizes of the clusters for 2≤ k≤10 cluster-solutions: # numbClusters=5; myClusters = sapply(2:10,function(numbClusters)table(cutree(hc, numbClusters))) names(myClusters) <- paste("Number of Clusters=", 2:10, sep = "") myClusters #To see which SubjectIDs are in which clusters: groups.10 <- cutree(hc, k = 10) sapply(unique(groups.10),function(g)data.1$\$$ID[groups.10 == g]) #To see which Treatments (Tx) are in which clusters: groups.2 <- cutree(hc, k = 2) sapply(unique(groups.2),function(g)data.1$\$$Tx[groups.2 == g]) # drill down deeper table(groups.2, data.1$\$$Tx) # For a small number of observations, we can often interpret the cluster solution directly by looking # at the labels of the observations that are in each cluster. # This is hard for larger data sets. To characterize clusters we can look at cluster summary statistics, # like the median, of the variables that were used to perform the cluster analysis broken down # by the groups that the cluster analysis identified.
The aggregate function will compute stats (e.g., median) on many variables simultaneously.
To look at the median values for the variables we've used in the cluster analysis, broken up by the cluster groups:
aggregate(data.1, list(groups.10),median) # may have to shrink data.1 prior to clustering! # data.2 <- data.1[,-c(1,3)] # Remove ID and Tx variables? aggregate(data.2, list(groups.2),median) # for only 2 clusters
Group | ID | Day | Tx | SelfEff | SelfEff25 | WPSS | SocSuppt | PMss | PMss3 | PhyAct | |
1 | 1 | 14 | 16 | 0 | 20 | -5 | -0.040 | 2.995 | 3.275 | 0.275 | 41 |
2 | 2 | 16 | 15 | 1 | 25 | 0 | 0.025 | 3.280 | 3.360 | 0.360 | 104 |
table(groups.2, data.1$\$$<u><b>PhyAct</b></u>) # library("Hmisc") describe(data.1$\$$PhyAct)
# It’s useful to add the numbers of observations in each group (aggregate returns a data frame, # that can be manipulated)
df.2 <- aggregate(data.1, list(groups.2),median)
data.frame(Cluster= df.2[,1], Freq=as.vector(table(groups.2)), df.2[,-1])
Cluster | Freq | ID | Day | Tx | SelfEff | SelfEff25 | WPSS | SocSuppt | PMss | PMss3 | PhyAct | |
1 | 1 | 570 | 14 | 16 | 0 | 20 | -5 | -0.040 | 2.995 | 3.275 | 0.275 | 41 |
2 | 2 | 330 | 16 | 15 | 1 | 25 | 0 | 0.025 | 3.280 | 3.360 | 0.360 | 104 |
Publications
- This paper examines nasal and bronchial tissue cultures as appropriate in vitro models for the assessment of smoking-induced adverse effects in the respiratory system (doi: 10.1177/1091581814551647), using “hclust” package. No data.
- This paper classified subtypes of gastric cancer based on epidemiologic and histologic and gene expression data. These new classifications of gastric cancer have implications for improving our understanding of disease biology and identification of unique molecular drivers for each gastric cancer subtype (doi: 10.1158/1078-0432.CCR-10-2203).
Repeat the clustering
# using centroids and squared Euclidean distance # cut the tree into 10 clusters and reconstruct the upper part of the tree from the cluster centers. hc <- hclust(dist(data.1), "cen") mem <- cutree(hc, k = 10) cent <- NULL for(k in 1:10){ cent <- rbind(cent, colMeans(data.1[mem == k, , drop = FALSE])) }
hc1 <- hclust(dist(cent), method = "cen", members = table(mem))
opar <- par(mfrow = c(1, 2)) plot(hc, labels = FALSE, hang = -1, main = "Original Tree") plot(hc1, hang = -1, main = "Re-start from 10 clusters") par(opar)
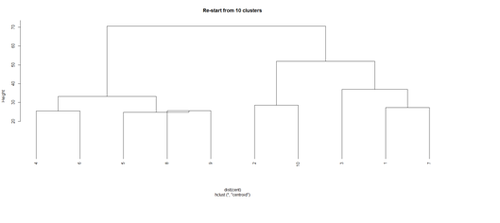
Identify subjects within each of the 10 classes
rect.hclust(hc, h=10)
# To save the cluster numbers to a new variable in the dataset, use the cutree function. # data.1$\$$clusterID <- cutree(hc, 10) data.1$\$$clusterID <- cutree(hc, 10) head(data.1)
ID | Day | Tx | SelfEff | SelfEff25 | WPSS | SocSuppt | PMss | PMss3 | PhyAct | CluserID | |
1 | 1 | 1 | 1 | 33 | 8 | 0.97 | 5.00 | 4.03 | 1.03 | 53 | 1 |
2 | 1 | 2 | 1 | 33 | 8 | -0.17 | 3.87 | 4.03 | 1.03 | 73 | 1 |
3 | 1 | 3 | 0 | 33 | 8 | 0.81 | 4.84 | 4.03 | 1.03 | 23 | 2 |
4 | 1 | 4 | 0 | 33 | 8 | -0.41 | 3.62 | 4.03 | 1.03 | 36 | 2 |
5 | 1 | 5 | 1 | 33 | 8 | 0.59 | 4.62 | 4.03 | 1.03 | 21 | 2 |
6 | 1 | 6 | 1 | 33 | 8 | -1.16 | 2.87 | 4.03 | 1.03 | 0 | 2 |
Phylogenetic tree diagram
# library("ape") plot(as.phylo(hc1), use.edge.length = TRUE, type = "fan") plot(as.phylo(hc), use.edge.length = TRUE, type = "fan", tip.color = hsv(runif(15, 0.65, 0.95), 1, 1, 0.7), label.offset = 1, cex = log(data.1$\$$ID, 10), col = "red") <center>[[Image:SMHS_SciVisualization36.png|500px]] </center> <center>[[Image:SMHS_SciVisualization37.png|500px]] </center> ==='"`UNIQ--h-20--QINU`"'Hands-on Activity (Health Behavior Risks)=== # load data CaseStudy09_HealthBehaviorRisks_Data data.2 <- read.csv("https://umich.instructure.com/files/399182/download?download_frd=1 ", sep=",", header = TRUE) # Classify the cases using these variables: "AGE_G" "SEX" "RACEGR3" "IMPEDUC" "IMPMRTL" # "EMPLOY1" "INCOMG" "CVDINFR4" "CVDCRHD4" "CVDSTRK3" "DIABETE3" "RFSMOK3" # "FRTLT1" "VEGLT1" data.raw <- data.2[,-c(1,14,17)] # Does the classification match either of these: # TOTINDA (Leisure time physical activities per month, 1=Yes, 2=No, 9=Don’t know/Refused/Missing) # RFDRHV4 (Heavy alcohol consumption, 1=No, 2=Yes, 9=Don’t know/Refused/Missing) hc = hclust(dist(data.raw), 'ave') # the agglomeration method can be specified "ward.D", "ward.D2", "single", "complete", "average" (= UPGMA), "mcquitty" (= WPGMA), "median" (= WPGMC) or "centroid" (= UPGMC) # (3) Plot clustering diagram par (mfrow=c(1,1)) # very simple dendrogram plot(hc) summary(data.2$\$$TOTINDA); summary(data.2$\$$RFDRHV4) cutree(hc, k = 2) # alternatively specify the height, which is, the value of the criterion associated with the # clustering method for the particular agglomeration -- cutree(hc, h= 10) table(cutree(hc, h= 10)) # cluster distribution # To identify the number of cases for varying number of clusters we can combine calls to cutree and table # in a call to sapply -- to see the sizes of the clusters for 2≤ k≤10 cluster-solutions: # numbClusters=4; myClusters = sapply(2:5,function(numbClusters)table(cutree(hc, numbClusters))) names(myClusters) <- paste("Number of Clusters=", 2:5, sep = "") myClusters #To see which SubjectIDs are in which clusters: table(cutree(hc, k=2)) groups.k.2 <- cutree(hc, k = 2) sapply(unique(groups.k.2),function(g)data.2$\$$ID[groups.k.2 == g])
#To see which TOTINDA (Leisure time physical activities per month, 1=Yes, 2=No, 9=Don’t # know/Refused/Missing) & whch RFDRHV4 are in which clusters: groups.k.3 <- cutree(hc, k = 3) sapply(unique(groups.k.3),function(g)data.2$\$$TOTINDA [groups.k.3 == g]) sapply(unique(groups.k.3),function(g)data.2$\$$RFDRHV4[groups.k.3 == g])
# Perhaps there are intrinsically 3 groups here e.g., 1, 2 and 9 … groups.k.3 <- cutree(hc, k = 3) sapply(unique(groups.k.3),function(g)data.2$\$$TOTINDA [groups.k.3 == g]) sapply(unique(groups.k.3),function(g)data.2$\$$RFDRHV4 [groups.k.3 == g])
# Note that there is quite a dependence between the outcome variables … plot(data.2$\$$RFDRHV4, data.2$\$$TOTINDA)
# drill down deeper table(groups.k.3, data.2$\$$RFDRHV4)
# To characterize clusters we can look at cluster summary statistics, # like the median, of the variables that were used to perform the cluster analysis broken down # by the groups that the cluster analysis identified. The aggregate function will compute stats # (e.g., median) on many variables simultaneously. To look at the median values for the variables # we've used in the cluster analysis, broken up by the cluster groups: aggregate(data.2, list(groups.k.3),median)
See Next
- Next See the Complex Network Visualization section
- Back to the Scientific Methods for Health Sciences EBook ToC
- SOCR Home page: http://www.socr.umich.edu
Translate this page: